You will find here some examples of works in progress which illustrate part of our research and knowledge dissemination activities.
Under construction forever !
PINNS for Table Tennis Balls Kinematics Identification
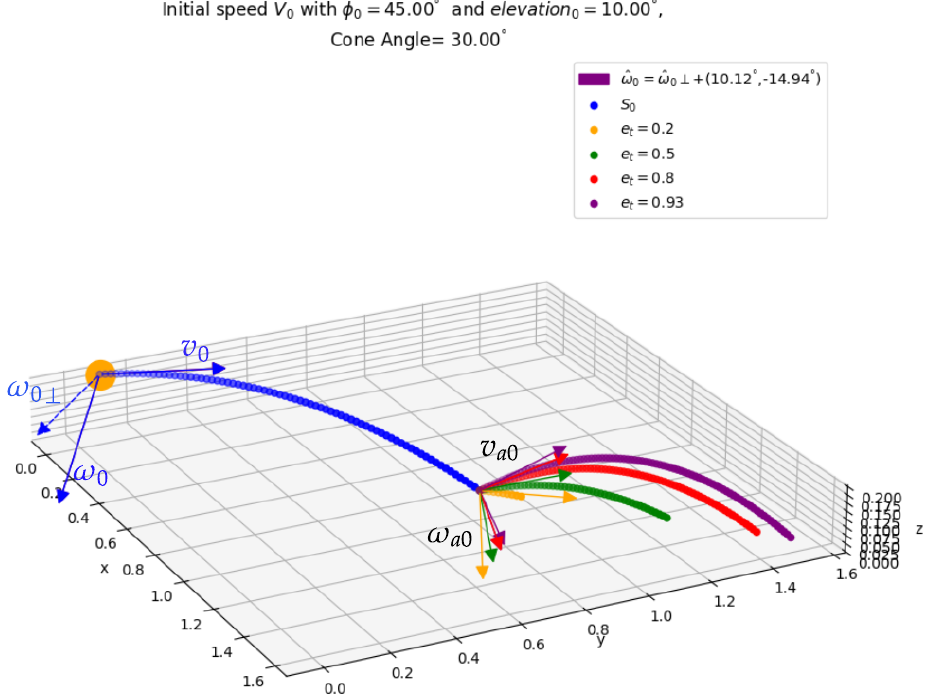
Vision-based methods for gesture analysis in sports can provide valuable insights to players and coaches, without any disturbing body-worn sensors. In table tennis, accurately predicting the trajectories of the ball and its 3D kinematics is crucial for assessing the effectiveness of a stroke. While several computer vision methods have investigated this area, they often focus on trajectory prediction solely, without considering physical parameters. Additionally, these studies are typically limited to controlled laboratory settings, which can be invasive and require extensive data collection. We propose a novel approach based on monocular vision video sequences relying on Physics-Informed Learning (PINN) to predict the 3D kinematics parameters using a small amount of data. Current advances involve taking account of rebounds through a set of PINNs linked together by physics equations.
Zaineb Chiha, Renaud Péteri, Laurent Mascarilla
Signal Processing: Image Communication - Elsevier, submitted
Zaineb Chiha, Renaud Péteri, Laurent Mascarilla
Proceedings of the International Conference on Content-Based Multimedia Indexing (CBMI), 2024
Zaineb Chiha, Renaud Péteri, Laurent Mascarilla
Actes du Congrès Reconnaissance des Formes, Image, Apprentissage et Perception (RFIAP), 2024
SAUMON : Suivi écologique temps réel de créAtures aqUatiques par iMagerie pour la prédictiOn de leur locomotioN
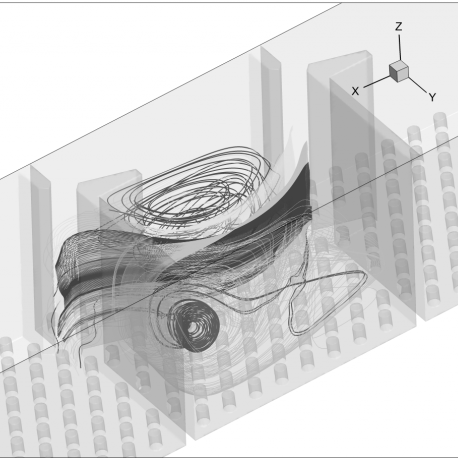
This project explores fish behavioral adaptation to flow conditions in fishways, a key topic in ecohydraulics. Although it's well known that water flow affects fish locomotion, the specific influence of flow features on behavior remains poorly understood. The Hydée team at the Pprime Institute uses experimental setups—a turbulent channel and a vertical-slot fishway—to study fish movement under controlled flow conditions.To analyze these behaviors, the project aims to develop advanced computer vision tools to detect fish, estimate 3D posture, and extract swimming features such as fin motion and body orientation. A large, annotated video dataset will enable the training of models to predict fish trajectories and assess fishway efficiency across species and conditions. In collaboration with XLIM and MIA laboratories, the project combines expertise in fluid dynamics, image analysis, and AI. It supports sustainable development goals and regional priorities on biodiversity and ecological transitions.
Benoit Tremblais, Damien Calluaud, Renaud Péteri, Laurent Mascarilla
Etudiant : Corentin Rabu (Université Bretagne Sud)